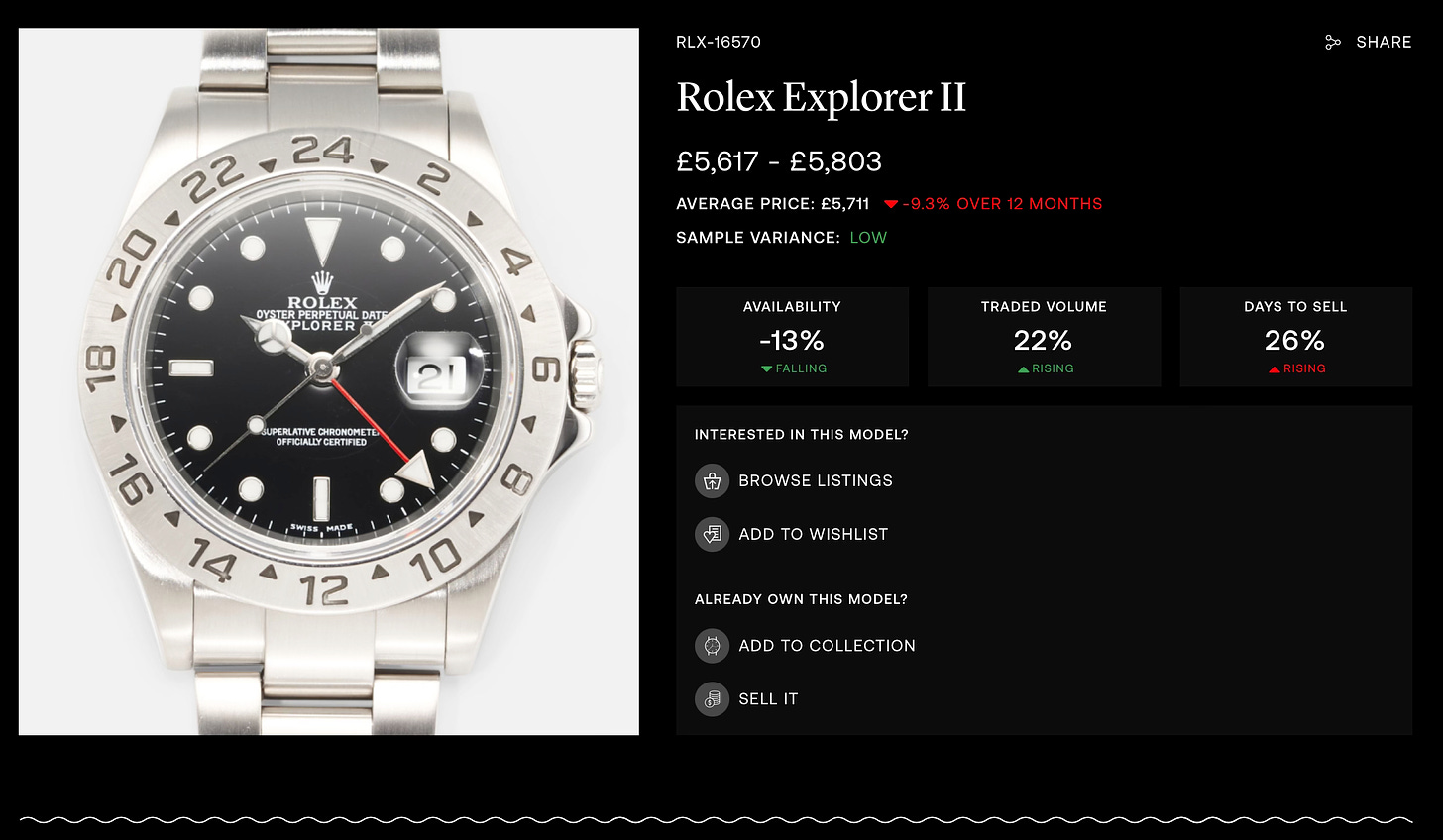
Up until about 5 years ago, watch pricing was primarily based on past transaction experience, verbal asks between professionals, and to a lesser extent public auction results. As an IWJG member, I also used to review the IWJG Price List (no longer being updated), which if I recall correctly was based on a survey of wholesaler transactions. Today, watch market data providers (hereon WMDPs) such as WatchCharts, WatchAnalytics, ChronoPulse, and Subdial publish coherent data and insightful information to the public. Not only do they provide price indexes, some WMDPs also provide valuation tools, which are usually based on the same data. I am infinitely thankful to and applaud these organizations and the hardworking people behind them that went through the painstaking process of capturing, cleaning, standardizing and presenting the data, especially given the hurdles of an old-fashioned and insular industry like ours.
The problem is, neither me nor anyone else I deal with uses this data for practical trading purposes. Don’t get me wrong, I do review them to get an overall idea of where prices are heading. But why am I not utilizing the price index data on a daily, operational basis?
In the diamond business, the weekly Rap Sheet is the Tower of Babel - everyone in the world uses it as a guide to actual pricing (% above or below Rap), so it’s very efficient as a starting point for negotiations. This is despite its opacity; it is based on asking prices on RapNet, but the methodology is not publicized, and is potentially adjusted manually by the publisher. This is in contrast to WMDPs’, whose data seems to be linked directly to actual transactions.1 So why aren’t we quoting off Chronopulse or WatchCharts already?
Obviously, there are some major differences:
Established in the 1970’s, the Rapaport Price List (RPL) is widely used among wholesale traders, with heavy inertia preventing a switch to other data providers.
RPL pricing is based on a standardized grading system, which is conducted by well-established 3rd party laboratories such as the GIA.
RPL is primarily used for diamonds traded as commodities, not finished goods. The latter would have higher heterogeneity, which are difficult to grade. Note that there is no Rap Sheet for colored precious stones, which have a larger number of parameters (heated, oiled, etc).
RPL is summarized into one table, allowing for quick and efficient negotiations.
In the US housing market, Zillow is one of the leading transaction data providers. The Zillow Home Value Index (ZHVI) today is so good that it can signal a turn in the market direction 6 weeks before the Case-Shiller Index. Zillow is also well known for their Zestimate automated valuation model (AVM), which allows users to get an estimate of the value of their homes. Importantly, the Zestimate is also the basis for calculation of the ZHVI. Like the Rap Sheet, the Zestimate pricing methodology is proprietary, but it seems to be an aggregated figure based on a hedonic price model, along with historic transaction and public tax record data. Zestimate is similar to the Chrono24 Appraisal Tool - both are AVMs, and unfortunately, both have not been widely adopted by professionals as benchmarks; rather, they are considered a “nice to have” data point by non-professional consumers and homeowners. Why?
AVMs such as Zillow are only up to date as the data behind them. Although improving as data accumulates, they are very sensitive to new listing and actual sale prices, often resulting in volatile swings in individual pricing estimates.
AVMs such as Zillow cannot fully account for repairs, upgrades, and changing local dynamics. They are also error prone in areas and for properties with low turnover rates. For watches, it would imply that pricing of rare iterations of watches would be inaccurate, social media preference trends would not be factored, and intrinsic heterogeneity such as replaced parts, incorrect accessories and nasty polishing, etc. are overlooked. Whereas they do pretty well when aggregated (ZHVI, Chronopulse, etc), the accuracy of individual valuations vary significantly.
Its not clear if AVM’s such as Zillow and Chrono24 accurately take into account actual and incoming supply in the market; perhaps Zillow is able to deduce some of this from permitting applications, but does Chrono24 help users adjust pricing based on the # of available listings of a particular watch? (Subdial and WatchCharts provide a handy supply monitor graph).
If I were to compare the current WMDPs to RPL or Zillow, I would say they are all generally closer to the latter, with a heavy emphasis on listing, hammer and/or actual sales data, organized by reference number.2
Now going back to my initial question, why am I and many other wholesalers not utilizing this data on a daily basis like the Rap Sheet?
Index and valuation price ranges and error rates are often higher than wholesaler margins
Too tedious and time-consuming for fast paced, live negotiations due to the sheer number of watch models
Due to variations in the discount or premium % off a retail price based index, it is generally more intuitive to use a wholesaler’s price index
Good old fashioned resistance to change
No one dominant provider like the RPL
Skepticism and stereotypes about data sources
# of references tracked are limited
Rarer or older references’ prices can be incorrect due to limited # of past trades
Does not account for new preference trends
Heterogeneity of finished goods such as watches makes pricing difficult and aggregate indexes less relevant at an operational or practical level
Before I go on, I would like to reiterate that I am throughly impressed by all the WMDPs that have completed the herculean task of compiling and presenting data in such a coherent manner. If any of the people behind those organizations are reading this, please take this as constructive feedback and note that I for one am very grateful for all your efforts and am looking forward to new developments.
Of the 10, I’m optimistic that 1-8 will naturally be solved over time as the data scales. While forecasts are impossible, I’m also confident that new AI-based models using data extracted from social media will ameliorate 9. Although there is a lot of skepticism about data sources, (for various reasons I will not get into here), widespread use should build confidence.
What is most difficult to solve today is 10. And this is the claim for underuse I hear most often - heterogeneity of watches (including differences in condition, design, materials and patina within a reference) is so great that it is impossible to accurately estimate values or build meaningful price indexes. Or in other words, watches are not commodities and so we should not expect to enjoy the productivity benefits of commodification. This seems to me to be a very hand-wavy, anti-progress and potentially Luddite claim to make, especially given that the aggregate indexes in many cases seem to correspond pretty well to reality. Without valuation tools and indexes, information asymmetry will remain high, increasing the risk premia of watches as an asset class. “Ask a reputable dealer” seems to me to be a very 20th century thing to advise, and ignores the increasing global fragmentation of our market.
Granted, it is an issue that is difficult to solve due to the sheer number of permutations. It may be less of an issue for newer watches in as-new condition, but as the years go by, heterogeneity generally increases, and with it the demand for price data adjusted for that heterogeneity.
The Chrono24 Appraisal Tool does take into account certain parameters (box/papers Y/N, dial color, condition VG, etc.), but they are still very basic.3 For example, let’s say I have a Rolex 16758 without its bracelet and a Cartier Tank Louis 78086 with a service dial. As most benchmarks of the 16758 include the bracelet, I would have to estimate or search for the value of a bracelet and back into the market price. For the Cartier I would have to separate/compile transactions or listings by dial type. This is tedious because the references numbers are the same, and hence requires a visual check of all the individual listings. And to complicate matters there is the problem of condition being hard to grade. How to price a cracked enamel dial? An over-polished case? In the old forum days there used to be a TZ grading system (who remembers?) but it was never very intuitive and fell out of use. Some dealers (usually with watchmaking backgrounds) use a replacement cost valuation method whereby they sum up the market value of all the major components, but this would require knowing the market value of those components.
Another thing to note is that despite our outwardly insistence on being data-driven, in practice people still take more stock in human-edited data like the Rap Sheet vs an algorithm or transaction data-based one like Zillow. Perhaps this reflects how much more confidence we place in human brains’ ability to holistically aggregate and correct data to make more sense in the real world. In the old days I remember trying to reconcile auction or retail prices for a watch with its corresponding price on the IWJG Price List - when I couldn’t reconcile them, I found myself mentally anchored to the IWJG’s human survey derived price - so I am part of the problem. This also reminds me of the controversial transition in finance from the human survey-based LIBOR rate to the transaction data-based (but more volatile) SOFR. It took a major global scandal in the early 2010’s for the industry to reluctantly switch to a transaction data based system a decade later.
So how to solve the heterogeneity problem without depending too much on humans? Let’s start with the theoretical future and work our way backwards.
In the future, a super hybrid valuation model would be composed of 3 parts4: a convolutional neural network (CNN) (a type of computer vision enabled AI), a hedonic regression submodel and an AI-enabled preference trend tracking submodel. A user would upload photos of say a Cartier Tank 78086 with a service dial and ask for a valuation. The CNN would be pre-trained on thousands of watch photos/videos and would be able to differentiate and identify an original dial vs a service dial. The CNN would then query the hedonic regression submodel, which would calculate the % discount applied in past sales transactions to a service dial vs an original dial. This submodel would also have many more parameters than we have now, including specific aspects of condition such as “Unpolished/Lightly Polished/Heavy Polish” and be able to discount for those parameters accordingly. Each listing could be automatically assigned a group identifier (similar to the Rolex guarantee code), which would be a combination of the reference number, extra parameters and condition/polish grade, etc. Based on this identification, it would spit out an accurate valuation with a 99% confidence interval, and form the basis for aggregate price index graphs. The CNN would also query an AI-enabled preference trend tracking submodel that analyzes social media chatter and influencers (and maybe # of watchers in sale or auction listings), to make a suggestion for a premium to market price for trending watches. By the time such a super hybrid model is up and running, humans would be more confident in AI models and may even prefer them over humans. It would be widely used by everyone around the world. The Tower of Babel for watches, and it would be quite impressive!
Unfortunately we are very far from this today. As I wrote here and here, ChatGPT (and other LLMs I also tested such as Gemini) had a lot of trouble with basic identification of watches, due to a lack of specific training and potentially even the inability to understand a 3D object in a 2D photo. The difficulty of identifying aftermarket or fake components from 2D photos is also a major issue. And most importantly, any model is only as good as its data - if you go through Chrono24 or any other platform, you will regularly find description errors or omissions (both by negligence and by choice) which would result in error rates beyond an acceptable threshold. So any attempt today to build a super hybrid model will involve another team of humans robotically annotating photos and descriptions of listings on platforms such as Chrono24, which is a lot of expensive grunt work that would never get done. And even if you did get this super hybrid model going today, what if nobody trusts something that is not human-reviewed and inherently volatile?
The temptation here is to go full human. Send out surveys to a pre-selected committee of wholesalers, dealers and representatives of each WMPD to edit a price chart of say the 200 most traded watches by condition. Strip out the outliers and publish a mean number for each. But in 2024, this seems to be a very backward and potentially corruptible5 way to solve a problem halfway.
My suggestion would be that in the interim, sale and auction platforms should transition to community-integrated platforms. They would more closely resemble the classic car auction site BringaTrailer.com, where both bidders and non-bidders actively comment and ask questions about listings. In other words, a merging of the current professional auction and sales platforms with the old forums. As watches are listed for sale, both enthusiasts and professionals would ask questions and add commentary on listings with errors or omissions. For example if a Cartier Tank Louis 78086 is listed, a commenter will ask the seller in the open commentary section whether there were any cracks in the dial, and the answer would be logged and associated with that listing. Sites such as Reddit have proven that commenters can be incentivized to participate with minimal financial reward, and even moderate and/or correct other commenters. After achieving some critical mass, the actual sales data along with its commentary would be accessible to an LLM. The LLM would be able to review thousands of listings, then parse and aggregate sales data and corresponding commentary for a specific criteria (for example a 78086 with crack-free dial). Unlike photos, text comments are cheaper to analyze, and would ameliorate the data quality/annotation problem at a much lower cost. Less likely, but it may eventually incentivize sellers to submit more thorough descriptions. And it would be the groundwork for a future CNN-enabled super hybrid model.
When I get lowballed for a 78086 at an IWJG show, I open a voice-enabled LLM wrapper app on my phone and ask, “What is the median market price for an unpolished or lightly polished Cartier 78086 with no cracks or damage in the dial, that comes with a deployant clasp?” The LLM would extract the sale data of those listings that fit my criteria based on the commentary and spit out a mean or median retail price, along with links to the actual listings. I would use this as ammo to quickly defend my price (key word: “quickly”). The LLM would also help me gauge supply in the market by counting the total # of listings that fit my criteria so I can estimate the correct % off retail.
To test my suggestion, I downloaded all current listings of Cartier 78086’s from Chrono24 into an excel file. I did visual checks of all 21 listings and added commentary such as “excellent dial”, “cracked dial”, “unpolished”, “heavily polished”, “deployant” or “d-buckle”, etc. I tried to make the comments haphazard - for some listings I wrote “nice all original example” while for others “excellent” or “dents on case otherwise good”. All this took me about 25 minutes to do6 . I then uploaded the file7 to Claude 3.5 Sonnet, and asked it the question in the screenshot below:
*UPDATE: (Please disregard the mean $9654 in the screenshot above, it was before I asked Claude to add 2 listings which it missed in the initial query)
Since Chrono24 prices are retail (I have no access to actual sale data), I estimated a 30%8 premium over the average market price, which I know is approximately $7500. So I expected an answer of approximately $9750. I also expected it to exclude the much more expensive listings with original box and papers. Below is the final output9:
Notice how Claude automatically stripped out the $15K+ outlier and suggested a median price of $9,938, which is probably a better measure than the arithmetic mean in most cases where there is a large spread in prices10. Its not in the screenshot, but the mean of the 6 listings is $9739, which is only $11 less or 0.1% off my expected price of $9750. Claude performed this analysis in a matter of seconds, which is crucial during negotiations.
The above is a very simple, non-technical solution, by no means perfect; I’m sure that the smarter people working at WMDP’s have much more robust, next generation-solutions for the heterogeneity problem. I for one am looking forward to them.
IDEX is the closest competitor of RPL. Supposedly their price list is automatically calculated based on actual transaction data on their platform. Rap is still king.
I’m assuming there is some adjustment (Heckman or some other method) to correct for unsold lots in the data.
The final valuation of the Chrono24 Appraisal Tool seems to be a simple arithmetic mean of some past sales transactions, which could be skewed either way depending on interpretation of condition.
I’m not an AI expert..this is all theoretical.
LIBOR had effectively been manipulated since the 1990’s.
I did not ask questions to sellers; eyeballing only.
I had to convert the excel to pdf format.
This is hard to guess unless you trade them often.
At first it missed 2 listings it should have included, probably due to the spreadsheet format, which LLMs seem to struggle with - this is why you see $9654 in the first screenshot. It is my understanding that LLMs perform better with plain text documents. Not in the screenshot, but I asked Claude to include those 2 listings for a total of 7.
Median is probably best for most cases, but in this case the parameters and prices were in a pretty tight range and there were only 21 listings to begin with, so I asked for the mean. Generally, people expect you to quote means, and when you say “median” they look at you kind of funny.
My experience applying large language models is similar to yours: the models exhibit poor accuracy out-of-the-box on watch-related tasks. But they perform _incredibly_ well at data analysis tasks, so I'm not surprised to see Sonnet 3.5 complete the task easily given some expert-curated listings.
Going a step further: while most LLMs cannot access the web, Perplexity can. Unfortunately the initial results aren't amazing, but web scraping is a solved problem (either with software or AI): https://www.perplexity.ai/search/please-pull-prices-for-cartier-1B.T1OMnSwe5u5xCZSzfJA
So there's certainly something here. It would be a short jump to incorporate forum listings via WatchCharts API access (https://watchcharts.com/api). One could even access the Chrono24 API *directly*, although I don't want to link those projects and risk taking them down.
Hi George, I left you a message on IG about this post. Mentioning it here in case you haven’t yet seen it.